Kuilin Zhang
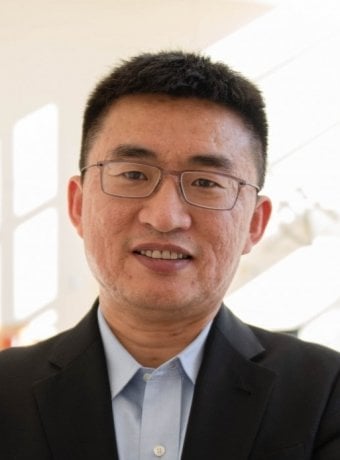
Contact
- klzhang@mtu.edu
- 906-487-1828
- Dillman 301i
- Associate Professor, Civil, Environmental, and Geospatial Engineering
- Affiliated Associate Professor, Computer Science
- PhD, Transportation Systems Analysis and Planning, Northwestern University
Biography
Dr. Zhang is Associate Professor in Transportation Systems and Computer Science. Dr. Zhang's research focuses on applying mathematical optimization, control theory, simulation analysis, game theory, machine learning, data science, and on-road vehicle testing to address safety, congestion, energy, environment, and resilience issues of critical civil infrastructure systems in Smart Cities. He advises PhD students from both CEGE and CS departments in his research group. Dr. Zhang received his PhD degree in Transportation Systems Analysis and Planning from the Department of Civil, Environmental, and Geospatial Engineering at Northwestern University in December 2009. After working as a Postdoctoral Fellow in the Transportation Center at Northwestern, he joined the Energy Systems Division at Argonne National Laboratory as a Postdoctoral Appointee in November 2010. He is a member of the Editorial Advisory Board of Transportation Research Part E - Logistics and Transportation Review, a member of Transportation Research Board (TRB) standing committees of Transportation Network Modeling (AEP40) and Freight Transportation Planning and Logistics (AT015), and a voting member for the Society of Automotive Engineers (SAE) Cooperative Automation Driving System (CADS) Committee. He is also a member of IEEE, INFORMS, and ITE. Dr. Zhang is a recipient of the NSF CAREER Award.
Links of Interest
Teaching Interests
- Transportation Network Analysis
- Traffic Flow Theory
- Travel Demand Analysis
- Transportation Systems Operations and Control
- Transportation Planning
- Traffic Engineering
- Optimization Methods
- Deep Reinforcement Learning and Model Predictive Control for Connected and Automated Vehicles
Research Interests
- Data-driven distributionally robust optimization (DRO) based control models for connected and automated vehicles (CAVs)
- Big traffic data analytics using machine learning
- Deep Reinforcement Learning (DRL) and Graph Neural Networks (GNN) for transportation applications
- Mobile and crowd sensing of dynamic traffic systems (e.g., Drone traffic monitoring, social media data for travel pattern analysis using Twitter and Foursquare smartphone apps)
- Dynamic network equilibrium and optimization
- Modeling and simulation of large-scale complex systems (e.g. Traffic Simulation)
- Freight logistics and supply chain systems (e.g., Intermodal Freight Modeling)
- Impact of plug-in electric vehicles to smart grid and transportation network systems
- Interdependency and resiliency of large-scale networked infrastructure systems
- Vehicular Ad-hoc Networks (VANETs)
- Smart Cities
- Cyber-Physical Systems